As I was travelling recently I took the book of Nick Bostrom with me, mainly because Elon Musk commented on it. The book is called Superintelligence. It purports to analyse the future development of intelligence in humans or machines, attempting to cast light on the way intelligence will increase and how artificial intelligence will present itself.
I was interested in this more or less state of the art view, because once I was aware of the state of the art in artificial intelligence, working on neuromorphic networks simulating the effects of substances like emotional neuromodulation using computers. I learned about robotics, machine learning, neurophysiology and read everything that anybody published. There’s no science I read in his book until now that I don’t know about, actually there is quite some that Nick doesn’t appear to know.
The question of how intelligence will develop, how human level intelligence will come about and how intelligence will rise as that point is reached are fascinating. They seem to be valid questions but to me Nick makes a number of shortcuts that make his analysis next to worthless. The most important ommision he makes is to define what intelligence is, or go into depth what an intelligence entails in the real world. Because he doesn’t analyse the way intelligence exists in our present world, nor proposes a definition of what intelligence is, he misses the opportunity to paint the alarming picture where it belongs, in the present, not at some point in the future.
_antiaircraft_xl.jpg)

The Microdomain of Logic
Nick starts with a review of past achievements in term of intelligence. His idea of intelligence here seems to be problem solving, like winning in a game. He states “Artificial intelligence already outperforms human intelligence in many domains” (p. 11) when applied to game playing computers. To me this statement has multiple flaws. First playing a game can count as goal oriented behavior, chess can be represented as a search though a tree graph of possible moves, the goal being to find the series of moves still left under the constraints created by the opponent where the computer wins. It is however usually extremely frail. It is an algorithm, meaning it can only work one way. One faulty wire, memory block or software statement and there is no more goal orientation. Saying that such kind of ‘intelligence’ surpasses human intelligence is wrong, because humans can be boxing and still play chess at the same time. Robustness is an obvious feature of the human brain, it uses many more neurons than necessary in every task and it ‘repairs’ itself.
With the different examples of problem solving and game playing discussed in Nicks review of the state of the art Nick adopts the complaint that as soon as a computer solves a problem considered intelligent it is no longer seen as intelligent (John McCarthy). Still without a proper notion of what intelligence is Nick guesses that natural language processing is a ‘AI-complete problem’. Here he borrows from the term ‘NP-complete’ or Nondeterministic Polynomial-Complete problems. These are problems that can’t be solved in polynominal time, meaning the duration necessary to solve them may explode with the problem size rendering them too time-consuming to solve ever. Cryptography uses NP-Complete algorithms to make sure noone can find a shortcut to decryption. It sounds cool to say ‘AI-complete’, to mean it requires human level AI to be doable, but it means very little. Phone answering systems do fine without it.
The Microdomain of language
Language is commonly considered to be a key aspect of artificial intelligence. The famous Turing Test for artificial intelligence consists of a human and a machine connected through teletype (precursor to chatting online) and communicating with the test subject, and if the test subject can’t notice the difference between the text from the human or the machine the machine has reached human intelligence. This view of what intelligence is obviously ‘symbolcentric’, something Turing’s mind certainly was. Concluding AI through this means will run in robustness issues really quick, and validity is limted by the test subject’s own intelligence. We can define intelligence in this case robust orientation towards correct or acceptable language, where ‘orientation’ of language comes down to selecting the words to use, like picking a route in a maze. We percieve language output as intelligence if it robustly succeeds in being correct (adequate to the conversation) or acceptable (socially) to the tester. There is no machine that has done this yet, quite a number of humans even struggle with it.
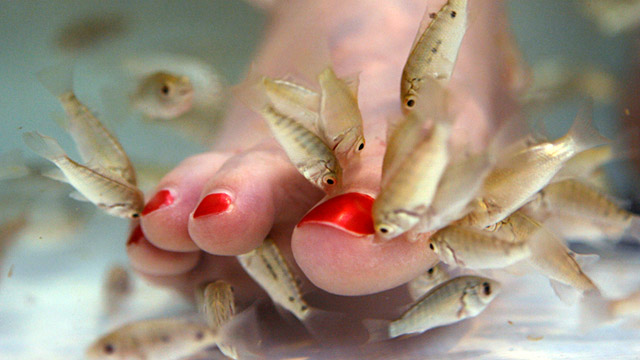
From First Principles
To understand intelligence as we posess it we should go back to the origins of it in the living world. The nervous system has a long developmental history first showing up in very tiny organism with a few of them, to eventually occur in humans, cows, elephants and chicken by the billions. The primary function of neurons is to make parts of the organism respond to something occuring in some other part. Neurons replace chemical diffusion, permeation of light or vibration penetrating to the part it connects to. Why? To quicken a response to threat or opportunity. Usually the goal of the response (which can be a construct of the observer or emergent) is to maintain homeostasis or survive.
Scales of AI
Simple organisms won’t be considered intelligent if human-level intelligence is used as a benchmark. Bentic organisms swim about in the sea and can find the light or darkness quickly, flee from stimuli but they can’t spell their name or solve puzzles. To me they are intelligent as they robustly orient towards their goals (stay in the dark may be one, stay away from disturbances which may eat them is another). The beauty is found in the fact the organism will be as intelligent as nature and its resources allow it to be. Now if the number of goals an organism can orient towards simultaneously increases we start to see a way to put these organisms on a scale with humans. (to be continued here, with a classification of ‘AI’)